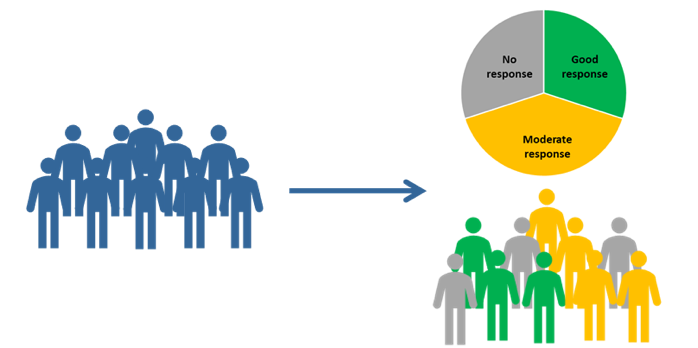
Continuous outcomes data from trials may be categorised using pre-defined cut-offs to generate ordered categorical outcomes data. Usually the percentage of patients who have improved by certain thresholds are reported in trial publications for all or just some of the pre-defined cut-off points. Examples of this type of categorical data include the European League Against Rheumatism (EULAR) and Psoriasis Area Severity Index (PASI) scales.
There are two main statistical approaches to modelling ordered categorical data in network meta-analysis (NMA):
- Analyse as binary data using an independent binomial logit model to estimate the treatment effects for each category separately
- Analyse as categorical data using a single multinomial probit model with a shared treatment effect across all categories
The binary approach requires fewer assumptions and the random effects model is easier to implement with this model (particularly important if significant heterogeneity is anticipated in the evidence network). However, this approach does ignore the correlation and natural ordering of the outcomes and therefore is not considered ‘efficient’ in terms of using all the information available. The categorical approach uses all available information, accounting for competing risks and the natural ordering of the outcome response categories. A key assumption of the multinomial probit model is the shared treatment effect; that is, the relative treatment effect is the same regardless of the response cut-off. It is essential that this assumption is deemed reasonable to pursue the second approach.
The two approaches both have their advantages and disadvantages. Therefore, it is important to understand the assumptions required for each model and their associated limitations before determining the most appropriate and robust analysis method for your data. Particularly when conducting NMA for health technology assessment, the rationale as to the choice of model and validation of the underlying assumptions of that model are essential.
For further details on the analysis of categorical outcomes in NMA or on any aspects of evidence generation contact us at info@mtechaccess.co.uk.
Click to read more about the author Sarah Batson